Apache StreamPipes saves the day when it comes to connecting to data sources in the IIoT world. Want to do more with your IIoT data than just analyze it in a dashboard? If so, this blog post is for you! We'll show you how to extract historical data from StreamPipes, use it to train a machine learning model, bring the model back to StreamPipes using ONNX, and apply the model to live data.
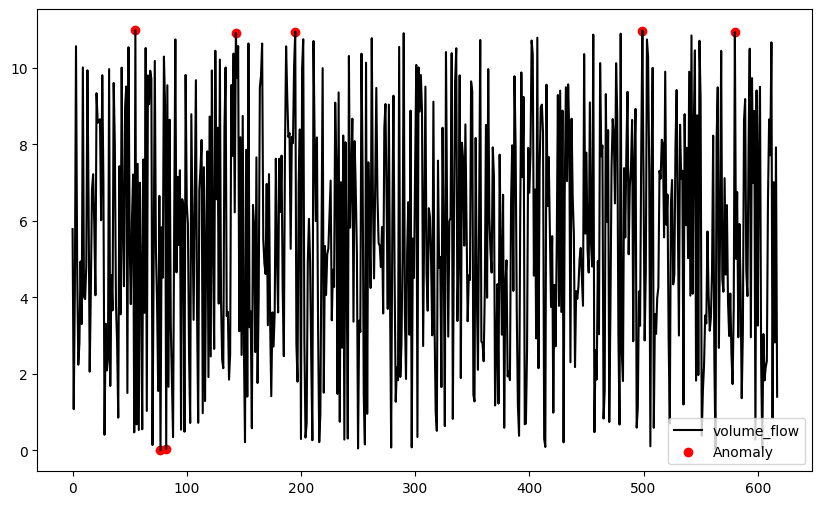
Motivation
With this blogpost we want to illustrate how one can easily extract historical IIoT data collected with StreamPipes, train a machine learning model on this data and bringing the model back to StreamPipes with the interoperability standard ONNX to make inference in live data.
A very common use case in the area of IIoT is the detection of anomalies, so we want to tackle this challenge in this
article as well. We will use data generated by
the Machine Data Simulator
adapter. More specifically, we will focus on the flowrate
data, which consists of various sensor values coming from a
water pipe system. Our goal is to keep an eye on the parameter volume_flow
, which represents the current volume flow
in
cubic meters/second. For this parameter, we want to detect anomalies that could indicate problems such as leaks,
blockages, etc.
To get the concerned data, we simply need to create an instance of the machine data simulator and persist the data in the data lake:
Set Up & Prepare Python Client
As a prerequisite, we need to install the StreamPipes Python client and all other dependencies,
pip install git+https://github.com/apache/streampipes.git#subdirectory=streampipes-client-python
pip install scikit-learn==1.4.0 skl2onnx==1.16.0 onnxruntime==1.17.1
The next step is to configure and initialize an instance of the client.
import os
from streampipes.client import StreamPipesClient
from streampipes.client.config import StreamPipesClientConfig
from streampipes.client.credential_provider import StreamPipesApiKeyCredentials
os.environ["BROKER-HOST"] = "localhost"
os.environ["KAFKA-PORT"] = "9094" # When using Kafka as message broker
config = StreamPipesClientConfig(
credential_provider=StreamPipesApiKeyCredentials(
username="admin@streampipes.apache.org",
api_key="TOKEN",
),
host_address="localhost",
https_disabled=True,
port=80
)
client = StreamPipesClient(client_config=config)
In case you have never worked with the Python client before and have problems to get started, please have a look at our tutorial.
If you already have an ONNX model and are only interested in applying it with StreamPipes on a data stream, you can skip the following section.
Model Training with Historic Data
As said above, the aim of our model is to detect anomalies of the volume_flow
parameter. For this task, we will use
Isolation Forests. Please note that the focus of the tutorial is not
on training the model, so please be patient even though the training is very simplified and lacks important preparation
steps such as standardization.
As a first step, lets query the flowrate
data from the StreamPipes data lake and extract the values of volume_flow
as a feature:
flowrate_df = client.dataLakeMeasureApi.get("flow-rate").to_pandas()
X = flowrate_df["volume_flow"].values.reshape(-1, 1).astype("float32")
As a next step, we can already train our model with the historic data:
from sklearn.ensemble import IsolationForest
model = IsolationForest(contamination=0.01)
model.fit(X)
The contamination
parameter models the proportion of outliers in the data. See
the scikit-learn
documentation for more information.
Here you can see how this simple model performs:
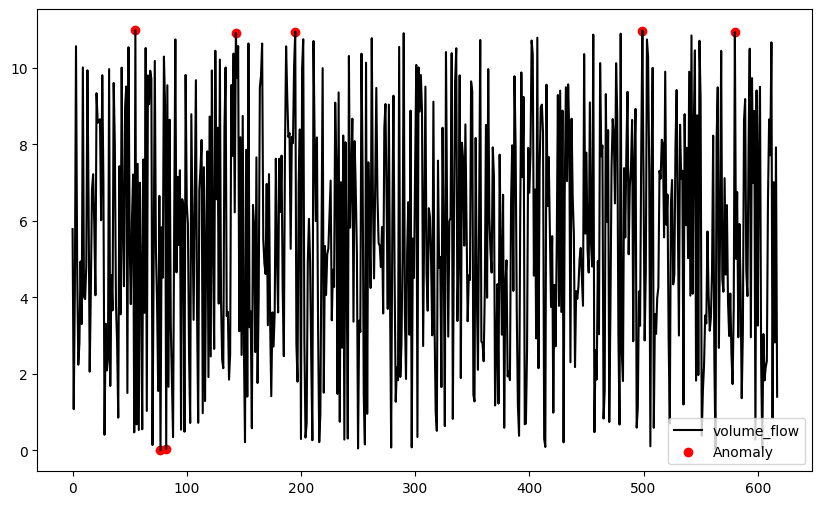
This doesn't look too bad, right? Let's continue by converting our model to the ONNX representation.
from onnxconverter_common import FloatTensorType
from skl2onnx import to_onnx
model_onnx = to_onnx(
model,
initial_types=[('input', FloatTensorType([None, X.shape[1]]))],
target_opset={'ai.onnx.ml': 3, 'ai.onnx': 15, '': 15}
)
with open("isolation_forest.onnx", "wb") as f:
f.write(model_onnx.SerializeToString())
Model Inference with Live Data
Utilizing a pre-trained model within StreamPipes becomes seamless with the ONNX interoperability standard, enabling effortless application of your existing model on live data streams.
Interacting with live data from StreamPipes is facilitated through StreamPipes functions. Below, we'll create a Python StreamPipes function that leverages an ONNX model to generate predictions for each incoming event, making the results accessible as a data stream within StreamPipes for subsequent steps.
So let's create an ONNXFunction
that is capable of applying a model in ONNX representation to a StreamPipes data
stream.
If you'd like to read more details about how functions are defined, refer
to our tutorial.
import numpy as np
import onnxruntime as rt
from streampipes.functions.broker.broker_handler import get_broker_description
from streampipes.functions.streampipes_function import StreamPipesFunction
from streampipes.functions.utils.data_stream_generator import create_data_stream, RuntimeType
from streampipes.functions.utils.function_context import FunctionContext
from streampipes.model.resource import FunctionDefinition, DataStream
from typing import Dict, Any, List
class ONNXFunction(StreamPipesFunction):
def __init__(self, feature_names: list[str], input_stream: DataStream):
output_stream = create_data_stream(
name="flowrate-prediction",
attributes={
"is_anomaly": RuntimeType.BOOLEAN.value
},
broker=get_broker_description(input_stream)
)
function_definition = FunctionDefinition(
consumed_streams=[input_stream.element_id]
).add_output_data_stream(output_stream)
self.feature_names = feature_names
self.input_name = None
self.output_name = None
self.session = None
super().__init__(function_definition=function_definition)
...
First, we need to take care about the data stream that is required to send the predictions from our function to StreamPipes. Thus, we create a dedicated output data stream which we need to provide with the attributes our event will consist of (a timestamp attribute is always added automatically). This output data stream needs to be registered at the function definition which is to be passed to the parent class. Lastly, we need to define some instance variables that are mainly required for the ONNX runtime.
Next, we need to ensure that ONNX runtime session is created on start up. Thus, we need to invoke an InferenceSession and retrieving the corresponding configuration parameters:
class ONNXFunction(StreamPipesFunction):
...
def onServiceStarted(self, context: FunctionContext) -> None:
self.session = rt.InferenceSession(
path_or_bytes="isolation_forest.onnx",
providers=rt.get_available_providers(),
)
self.input_name = self.session.get_inputs()[0].name
self.output_name = self.session.get_outputs()[0].name
...
Lastly, we need to implement the inference logic that is applied to every event.
If you have brought up your own model, you need to adapt line 10-13
:
class ONNXFunction(StreamPipesFunction):
...
def onEvent(self, event: Dict[str, Any], streamId: str) -> None:
feature_vector = []
for feature in self.feature_names:
feature_vector.append(event[feature])
prediction = self.session.run(
[self.output_name],
{self.input_name: np.expand_dims(np.array(feature_vector), axis=0).astype("float32")}
)[0]
output = {
"is_anomaly": int(prediction[0]) == -1
}
self.add_output(
stream_id=self.function_definition.get_output_stream_ids()[0],
event=output
)
def onServiceStopped(self) -> None:
pass
Having the function code in place, we can start the function with the following:
from streampipes.functions.registration import Registration
from streampipes.functions.function_handler import FunctionHandler
stream = [
stream
for stream
in client.dataStreamApi.all()
if stream.name == "flow-rate"
][0]
function = ONNXFunction(
feature_names=["volume_flow"],
input_stream=stream
)
registration = Registration()
registration.register(function)
function_handler = FunctionHandler(registration, client)
function_handler.initializeFunctions()
We can now access the live values of the prediction in the StreamPipes UI, e.g., in the pipeline editor.
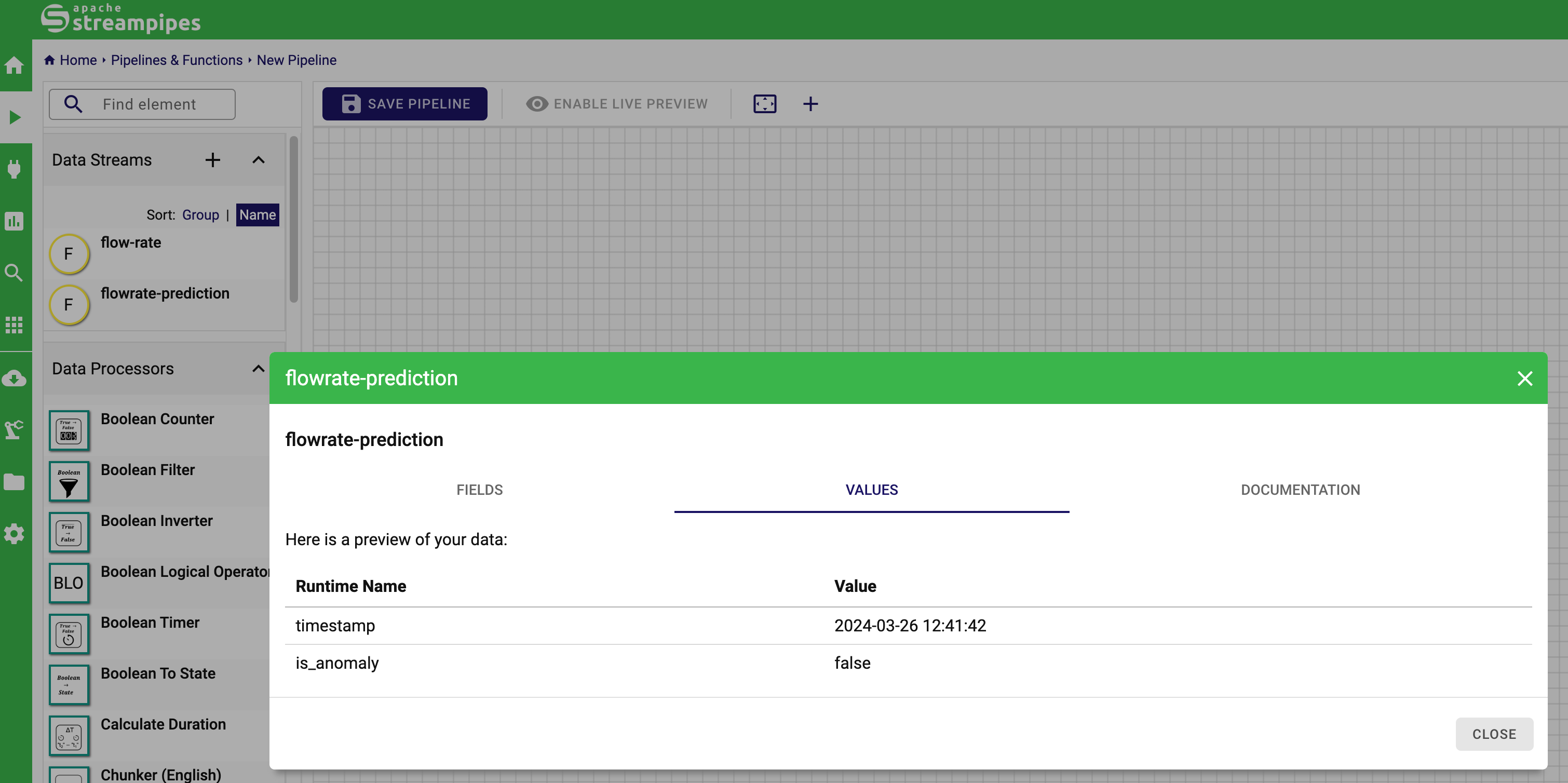
From here on you can further work with the prediction events in StreamPipes, e.g., by sending notifications to MS Teams.